Particle agglomeration analysis using PATVIS APA and deep learning
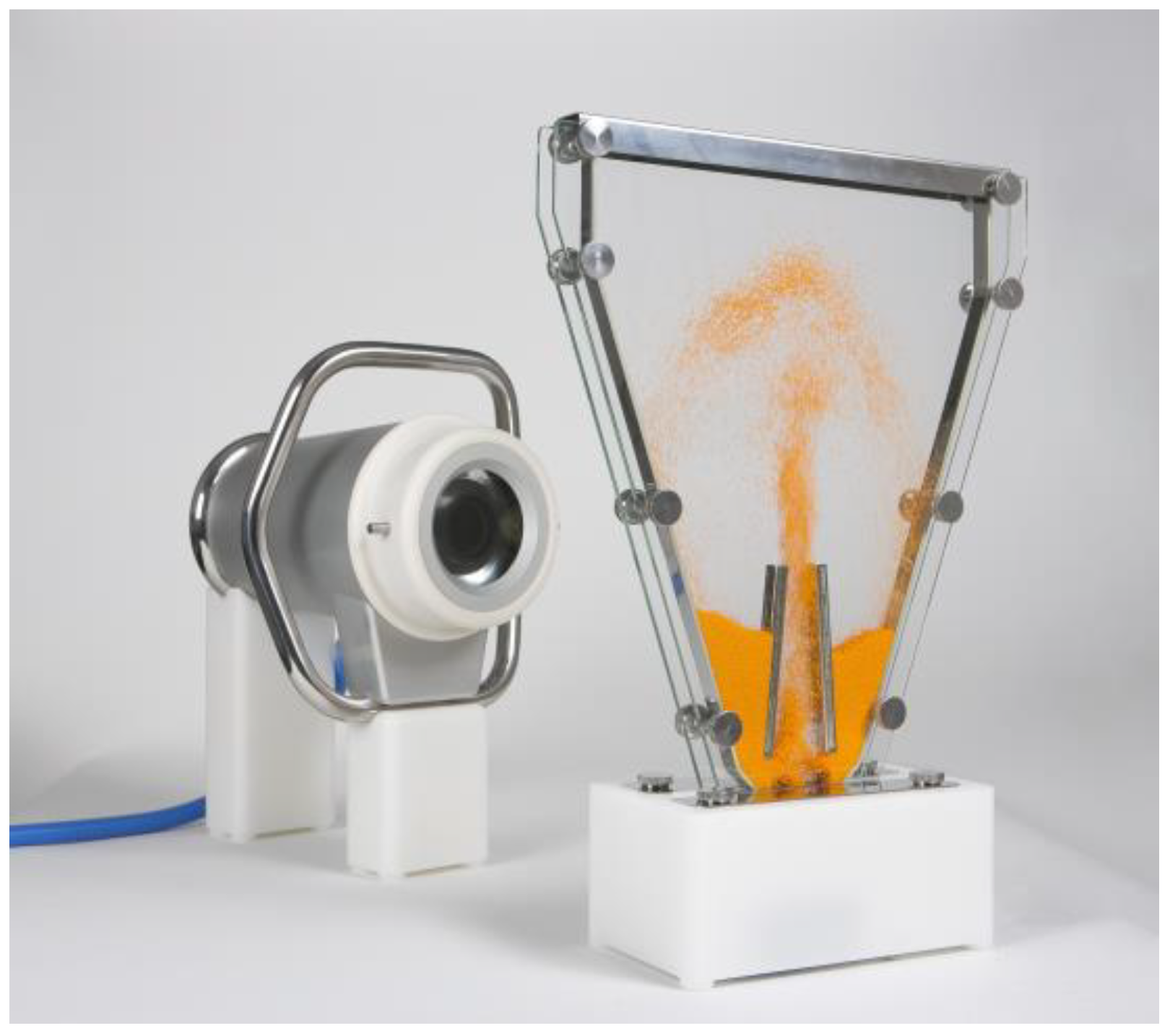
Introduction: Pharmaceutical, chemical, cosmetic, food, and other industries utilize various processes to modify particle properties, where agglomeration of particles occurs either as an accurately controlled process or as an undesired phenomenon. In both cases, real-time estimation of the particle agglomeration provides means for process monitoring and process control.
In pellet coating, agglomeration negatively affects the process yield and the coat integrity. Variations in the properties of materials and operating conditions as well as sporadic events are major causes for unwanted agglomeration. Traditionally, the agglomerate fraction is assessed at the end of the coating process by sieve analysis, which is an approach with obvious drawbacks. Currently, the most promising in-line agglomerate fraction analysis methods applied to pellet coating processes are based on optical measurements [1].
In this paper, we present a novel image analysis approach for automatic, non-invasive recognition of agglomerated particles and estimation of the agglomerate fraction. Image sequences of mixtures of individual pellets and agglomerates with predefined ratio (reference agglomerate fraction) were acquired and the detected particles were classified. Furthermore, the agglomerate fraction of each mixture was assessed and compared to the reference values.
Download the full article as a PDF here or download the related poster here
More about microcrystalline cellulose
Conclusion: An automated image analysis method was developed for in-line recognition of agglomerates and estimation of the agglomerate fraction of pellets during the coating process. The obtained results compared to the previously proposed method [3] indicated a considerably improved recognition of agglomerates, which consequently improves the accuracy of the agglomerate fraction estimation.
Capturing images in controlled conditions separately for individual pellets and agglomerates facilitates the acquisition of learning images and is a key step for the practical feasibility of using machine learning to identify agglomerates in actual pellet coating processes.
Eventually, the timely information about the agglomerate fraction can be used for process control by manual or automatic optimization of process parameters to retain the agglomerate fraction in an acceptable range.
Although we tested the proposed method only on pharmaceutical pellets, it can be used for agglomerate fraction estimation of particles in other processes as well.
Article information: A. Mehle, D. Kitak, G. Podrekar, D. Tomaževič. Sensum, Computer Vision Systems, Tehnološki park 21, 1000 Ljubljana, Slovenia, [email protected]. Laboratory of Imaging Technologies, Faculty of Electrical Engineering, University of Ljubljana, Tržaška cesta 25, 1000 Ljubljana, Slovenia.